
dtype, scope = scope, data_alignment = 32, offset_factor = 256 ) def intrin_func ( ins, outs ): ib = tvm. compute (( n, n ), lambda i, j : A, name = "C" ) BC = tvm. dtype, scope = "shared", data_alignment = 32, offset_factor = 256 ) C = te.

placeholder (( n, n ), name = "A", dtype = "float16" ) BA = tvm. compute_inline ()ĭef intrin_wmma_load_matrix ( scope ): n = 16 A = te. astype ( "float32" ), axis =, ), name = "Conv", ) s = te. compute ( output_shape, lambda n, h, w, o, nn, oo : te. all ( h >= pad_h, h - pad_h = pad_w, w - pad_w < width ), A, tvm. compute ( ( batch_size // block_size, height + 2 * pad_h, width + 2 * pad_w, in_channels // block_size, block_size, block_size, ), lambda n, h, w, i, nn, ii : tvm. placeholder ( kernel_shape, name = "W", dtype = "float16" ) Apad = te. placeholder ( data_shape, name = "A", dtype = "float16" ) W = te. reduce_axis (( 0, block_size ), name = "ii" ) # Algorithm A = te. reduce_axis (( 0, in_channels // block_size ), name = "ic" ) ii = te.
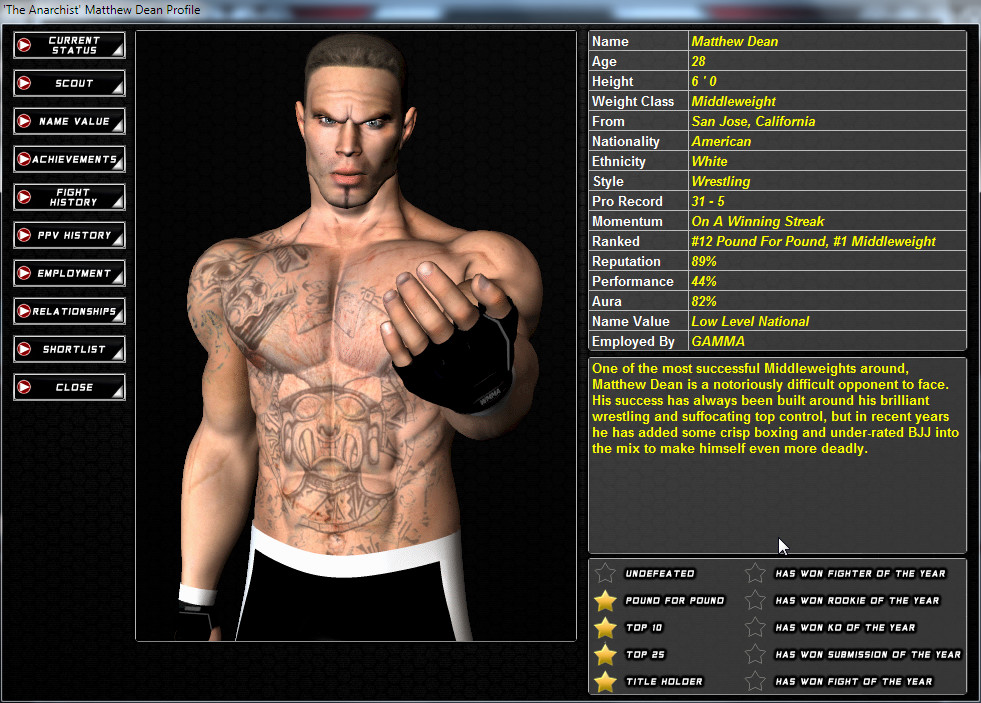
reduce_axis (( 0, kernel_w ), name = "kw" ) ic = te.
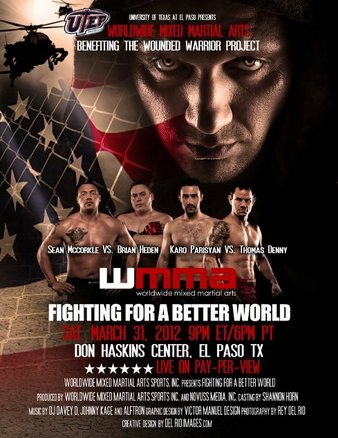
reduce_axis (( 0, kernel_h ), name = "kh" ) kw = te. Import tvm from tvm import te import numpy as np from tvm.contrib import nvcc # The sizes of inputs and filters batch_size = 256 height = 14 width = 14 in_channels = 256 out_channels = 512 kernel_h = 3 kernel_w = 3 pad_h = 1 pad_w = 1 stride_h = 1 stride_w = 1 # TensorCore shape block_size = 16 assert batch_size % block_size = 0 assert in_channels % block_size = 0 assert out_channels % block_size = 0 # Input feature map: (N, H, W, IC, n, ic) data_shape = ( batch_size // block_size, height, width, in_channels // block_size, block_size, block_size, ) # Kernel: (H, W, IC, OC, ic, oc) kernel_shape = ( kernel_h, kernel_w, in_channels // block_size, out_channels // block_size, block_size, block_size, ) # Output feature map: (N, H, W, OC, n, oc) output_shape = ( batch_size // block_size, height, width, out_channels // block_size, block_size, block_size, ) # Reduction axes kh = te. Use AutoScheduler for Template-Free Scheduling.How to optimize convolution using TensorCores.Work With Tensor Expression and Schedules.
